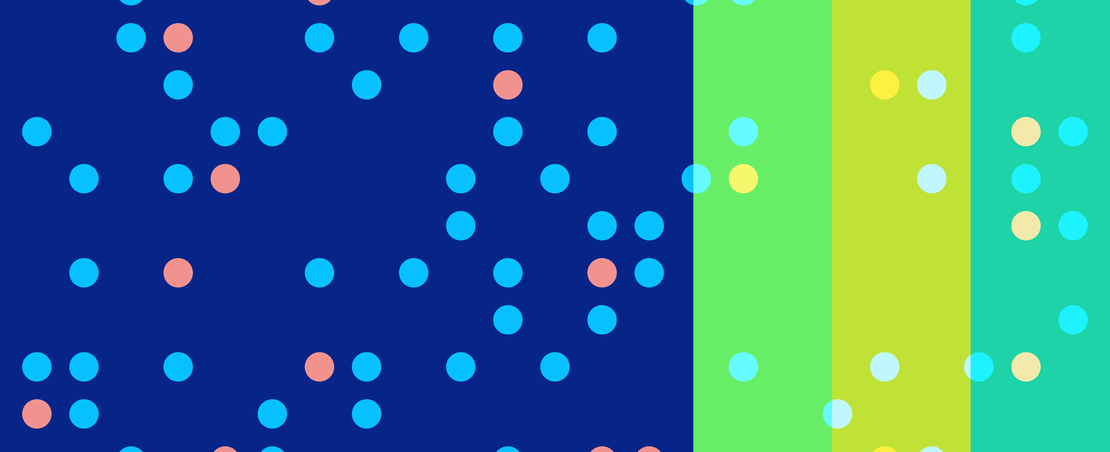
In 2023, AI was the word (and Collins Dictionary Word of the Year). Although AI research has experienced surges and lulls (‘summers’ and ‘winters’) in its development since the 1950s, the launch of OpenAI’s ChatGPT in November 2022 - gave AI rizz (Oxford Dictionary’s 2023 Word of the Year) like never before. ChatGPT was one of many chatbot and AI-powered auto-generative text and image systems, able to produce human-sounding replies and engage in human-like interactions when prompted.
Generative AI has a variety of potential applications. It has delighted students with its near-instantaneous ability to produce an essay on any subject, confused everyone with 6-fingered images of people, and launched a thousand X posts on [p]DOOM - the probability of AI ending human life as we know it. We set out to see whether it could assist with one of the more arduous tasks that face researchers, activists, grant writers and a whole host of people who rely on data to support and enable their work - finding and utilising appropriate datasets.
The ODI has been working in collaboration with King’s College London to answer these questions. We’re thinking about how people can better participate in the data economy - this project focuses on enabling participation in data use. In our work we’ve found that participation often occurs earlier on in the data lifecycle, particularly in the way that data is collected and governed, and there are less examples of ways in which people are empowered to use and share data. The development of AI presents new opportunities in this space, and this research seeks to explore the potential of these models to empower people to find and use data. In this paper, ‘Prompting Datasets: Data Discovery with Conversational Agents’, we identify emerging practices in data discovery and assess the pros and cons of using these models for data discovery.
What did we do
To explore how users might engage with generative AI models, we invited three groups of participants with a range of technical ability to take part in three workshops. The workshops involved asking them to use generative AI chatbots based on large language models to find datasets online and to explain what the dataset contained. These sessions took place in London and Berlin (two in English and one in German), and each used a different generative AI tool - ChatGPT 3.5, ChatGPT 4 and Bard. The participants answered a survey before and after the session to assess their understanding and experience of generative AI, and their reflections on their utility. The participants also spent some time discussing their hopes and expectations for these tools before testing them. The results emerged from a qualitative analysis of the prompt transcripts, and a quantitative analysis of the survey responses.
What did we find out
Did our users like conversational search via the LLMs they tested? Did they find it effective? Interestingly, most enjoyed the experience of using it, even if many agreed that as search, it is inferior to current web options such as Google. This was largely due to a failure to offer links (even when the GPT was web-enabled) and also because users did not necessarily have complete confidence in the data sources. A purported benefit of conversational search is that users can explicitly define their motivation for search. Other research suggests that telling a generative AI to play a specific role, or pick a ‘persona’, creates better results. Our users did not specify why they were carrying out their search as part of the prompts. This may be because when we use web search, we are used to thinking of our search motivation as implicit, rather than explicit. This suggests this may be a skill users need to acquire. However, a real benefit our users found was that looking for and exploring data with generative AI chatbots meant that further tasks - such as generating code to access an API or creating a graph - can be integrated into the conversation. This feature is a real differentiator with conventional search.
Looking at how our users searched for, and made sense of data, we came up with the following set of preliminary guidelines for prompting, in addition to using a persona (you can read more about using personas for prompting here):
- Select the correct prompt format for the data: Writing the name of a dataset in the prompt is most likely to return the requested dataset without much supporting information (unless this is specified in the prompt); describing a dataset is likely to return suggestions of datasets with some supporting information and implying a dataset (where data is requested, but the word ‘data’ is not used in the prompt) is likely to return a narrative description of some key data points.
- Mix request prompts for more effective discovery: The different types of requests can be employed iteratively, in order to build a better picture of the data. For example, a user might begin with an ‘implied’ prompt in order to understand the bigger narrative of a dataset, and then move to a ‘described’ prompt in order to explore more about the data itself.
- Use results as a base for improvement: It is common for users to request generative AI chatbots to supply a draft of a text, such as a letter or report, which they then edit and improve. Similarly, the results of data discovery can be used as a basis for further search, by continued prompting or additional search via traditional means.
- Use reminders: The generative AI chatbot responds to the prompts of the users but is fundamentally passive. Building towards the goal should include explicitly reminding the generative AI chatbot of the task and previous interactions in the conversation - eg, ‘earlier, you suggested creating a graph…’.
- Exploit the coding abilities of generative AI chatbots: For instance, instead of asking for a visualisation, ask for code to create a visualisation. An incorrect visualisation with no explanation of how it was created is harder to debug than incorrect code.
Overall, working with our users led us to six areas of search where we think generative AI chatbots offer the possibility of a real improvement over current data discovery processes, and four areas where there are still substantial problems.
On the promise side, generative AI chatbots perform curation of datasets, presenting a limited number of focused results. This has real promise for user-centric data discovery. We also noted that sometimes curation took the form of creating ‘micro’ datasets from unstructured text. When working with data, ChatGPT and BARD also provided useful supplemental information, such as reminding users to always check the terms of the licence associated with the data – which can improve data literacy. Similarly, it can also provide advice on how to use the data. On the other hand, generative AI chatbots can also incorporate feedback from the user to shape their output. We’re also excited about how generative AI chatbots can facilitate managing complex queries, such as being able to search for and book an entire holiday in one session rather than across multiple web searches. The promise that holds the most potential for improving general data usability is that of multimodality – that it can provide output as code, text summaries, tables, graphs and more.
Of the challenges, the most well-known of these is the tendency of generative AI chatbots to hallucinate. In the workshops, fictitious reports, graphs and sources were all given to users. Related to this is inconsistency - generative AI chatbots returned different results even when users entered exactly the same prompts. The generative AI chatbots also were poor at offering explanations for the results and information they presented. The last challenge was the expectation gap. Users need to approach conversational dataset search less in the anticipation that they will receive a magic bullet answer, and more in the expectation of receiving support to find and use an appropriate dataset, which may happen using a different tool on the web.
The findings of this research indicate the potential that large language models have in supporting dataset discovery, as well as the main drawbacks. In their current form, we found that generative AI chatbots are likely to be useful supporting tools, as a way to start your search on a new topic due to the conversational tone which you can take, and their ability to curate data and information drawn from multiple sources. However, they are currently not to be relied on, and each result should be double checked via traditional means. Going forward, and with continued research, generative AI models will improve on their reliability. Many are already being connected to search engines to improve their accuracy, such as Bing, but this research demonstrates that these models are still a work in progress in regards to validity.
The world of AI continues to evolve rapidly, both the number of models and the capabilities of these models have grown in the last year. At CES 2024, AI took over the show with lots of attention going to Rabbit AI, a personal assistant that can navigate both the physical and online worlds, based on a Large Action Model (LAMs are capable of understanding any sort of user interface and navigating through it just like a human being). Crucially, our research found that users enjoy interacting with conversational agents - so much so that the user experience often makes up for the deficit in actual results. This means that it is likely the technological developments that are set to continue in the coming months and years will gain traction. These models will impact the way we use technology for search, and will require new methods to evaluate them, new datasets to compare solutions, and a better understanding of users needs to maximise the benefit of these technologies.
What happens next
Many people are excluded from participating in the data economy due to a lack of skills or awareness about technology, this phenomenon is known as the digital divide. We’re excited about the potential of generative AI models to reduce the barriers to entry for using technology, enabling people who are currently excluded to take part. However additional work is needed to understand the promise and challenges of these systems as they develop to ensure they meet the needs of the digitally excluded. Research in this area is moving fast, and in many topics, for example, King’s College London also hosted a hackathon for knowledge engineers to engage with the potential of LLMs.
Get in touch if you’re working in this space, or would like to be involved in future research or learn more about the potential of generative AI in bridging the digital divide. We will be hosting data prompting workshops in February 2024 and a Hackathon later in the spring with Microsoft to answer some of the questions raised by this research - let us know if you’re interested.