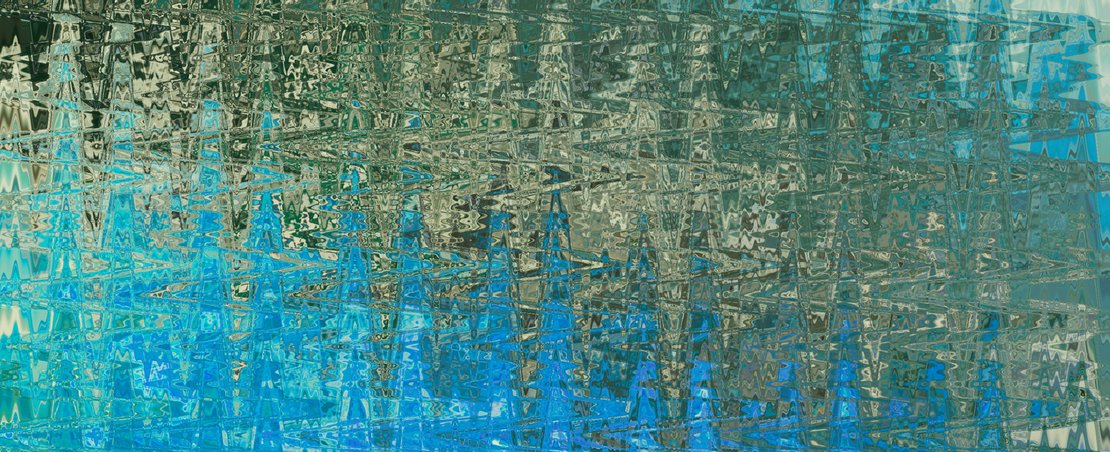
In this blogpost, Dr Konrad Kollnig and Calum Inverarity consider some of the legal and regulatory obstacles that remain to the adoption of PETs at present. This article does not aim to provide legal guidance. If you need such, you should always consult a lawyer. Modern privacy-enhancing technologies (PETs) and confidential computing approaches – like federated learning or secure multi-party computation – are increasingly popular. Rather than just make data-processing more privacy-preserving, organisations also hope to employ them in order to help compliance with data protection and privacy laws, like the General Data Protection Regulation (GDPR) in Europe and the California Consumer Privacy Act (CCPA) in California. When and whether modern PETs actually help with legal compliance is, however, still unclear. European data protection authorities have so far provided limited clarification on how modern PETs may help with GDPR compliance (recent draft guidance by the ICO is a notable exception). Crucially, the legal analysis depends much on the specific technology used, since PETs is an umbrella term for a wide range of different approaches. To bring some light to the debate, the following blog article will discuss a range of common legal obstacles in the PETs domain, especially in the context of Europe’s GDPR, and aims to elucidate when PETs may not help with compliance and on what aspects more guidance and clarification would be needed.
GDPR, anonymised data, and machine learning
One of the most important PETs does not traditionally fall into this category but shares some important features, the training and use of machine learning algorithms – commonly referred to as Artificial Intelligence (AI). These methods usually try to learn a statistical model based on input data. When the input data is personal data (i.e. any information that relates to a person), then the training process involves the processing of personal data and the obligations of the GDPR apply. Conversely, if the data in question is not personal, then GDPR does not apply. However, the line between personal and non-personal is not always clear and needs to be considered extremely carefully. Often, in the context of PETs, an organisation might train machine learning models on allegedly ‘anonymised’ data. If there is reason to believe that someone can turn ‘anonymised’ data back into personal data and learn data about individuals, then the obligations of the GDPR apply to the use of this supposedly ‘anonymised’ data. Crucially, GDPR obligations always apply when personal data is processed, even when applying ‘anonymisation’ techniques to personal data. Only if data is truly anonymised, GDPR does not apply. A similar argument applies to trained machine learning models. If these are trained on personal data, then the model weights will likely contain the data that this model was trained on. In this case, the use of these trained machine learning models may also constitute processing of personal data, in which case the obligations of the GDPR would apply. To complicate matters further, it has been demonstrated that personal data can sometimes be reconstructed from trained neural networks (also known as a model inversion attack). Thus, it is important to assess continuously over time how to protect personal data (even if it seems to be ‘anonymised’), as attacks on machine learning models develop and our understanding of modern technologies changes. In the USA, an important related case is currently pending before the courts. In this case, Getty Images alleges that Stability AI infringed on copyright law in training their image generation software ‘Stable Diffusion’. However, it remains difficult to reconstruct input images from the Stable Diffusion system, and therefore to prove that copyrighted material has been used. This case – and similar related cases – will have important implications for the legal obligations in the use of machine learning techniques, particularly the level of evidence that is required to bring forward court cases concerning data-reliant systems.
The issue of controllership
Organisations commonly work with different organisations when training machine learning models. One of the most promising approaches to support this is federated learning, a machine learning technique pioneered by Google. Federated learning enables the training of machine learning models across different computer systems without ever sharing that data directly between those computer systems. Since data is never shared, federated learning is commonly referred to as a PET. As we discussed in our recent research, this approach does usually not so much provide privacy and compliance benefits rather than others, such as increased efficiency of training. If personal data is processed in a federated learning application, there are two important cases under the GDPR: cross-silo and cross-device training. Cross-device training is commonly used by large tech companies, like Google and Apple, to train machine learning models across the millions of devices used by consumers of their products. Since these end-users usually don’t determine the ‘purposes and means of the processing of personal data’, the exclusive responsibility would lie with the tech companies and the obligations of being a ‘data controller’ under the GDPR would most likely apply to them. In the case of cross-silo FL, different organisations, e.g. hospitals that seek to develop new treatments, choose to collaborate on training machine learning models. Depending on the level of involvement of the participating organisations, the obligations for the data processing might then be shared across those organisations and they may all classify as ‘data controllers’. A particularly interesting case arises when third-party software is used for the development and deployment of PET systems. According to evolving case law by the Court of Justice of the European Union (CJEU) on the interpretation of the GDPR in practice, the responsibility of software development, deployment and use might be shared across large numbers of organisations, according to their level of involvement; this case (IAB Europe v Gegevensbeschermingsautoriteit) has, however, not yet been decided by the court. The underlying reasoning is based on previous CJEU case law on the topic of joint controllership (e.g. Unabhängiges Landeszentrum für Datenschutz Schleswig-Holstein v Wirtschaftsakademie Schleswig-Holstein GmbH) that found that organisations may be classified as controllers, even if they never have access to any personal data, and that each organisation may similarly bear the responsibilities of controllership for its part in the data processing chain. Through these rulings, the CJEU is steadily addressing the challenge that modern software systems usually consist of a large number of stakeholders, each of which makes their own contribution. This also underlines the potential complexity in assessing the responsibilities when parties collaborate, e.g. on federated learning, multi-party computation (MPC), or other collaborative PETs. While the UK withdrew from the CJEU in December 2020, most case law on joint controllership remains applicable since it was issued before the withdrawal date.
Fulfilling the GDPR’s principles
Another challenge, under the GDPR, relates to the use of cryptographic methods – the core of many PETs, such as homomorphic encryption or synthetic data – in the processing of data. Article 5 of the GDPR specifies seven principles that the processing of personal data must balance and uphold:
- Lawfulness, fairness and transparency
- Purpose limitation
- Data minimisation
- Accuracy
- Storage limitation
- Integrity and confidentiality
- Accountability
The use of cryptographic methods tends to help fulfil some of these principles, but may also impede others. Many PETs help address ‘integrity and confidentiality’ by making it more difficult to obtain the raw data, thereby helping the security of data processing. As for the other principles, some PETs may actually make compliance with them harder. For example, PETs tend to work against ‘lawfulness, fairness and transparency’ because cryptographic methods – by definition – aim to reduce insights into operations for a maximally large number of bystanders. To illustrate this further, the mitigation of bias remains a big challenge in the deployment of federated learning, since access to the raw data is limited. This might be okay if the use of PETs overall creates a lot of good, but one would usually expect that the data controller balances out the different interests at stake (such as reasonable commercial interests vs deficits in terms of bias) by providing other appropriate safeguards. PETs may also run counter to ‘accountability’, which obliges controllers to be able to prove compliance with the other six principles. Less accountability might also encourage the infringement on ‘purpose limitation’ and the use of data beyond the initially intended scope and purpose (e.g. through ‘mission creep’). While the ICO argues in their draft guidance that PETs can help organisations fulfil the principle of ‘data minimisation’, PETs may also run counter the ‘data minimisation’ principle since they principally aim to enable greater – rather than less – data sharing and may thus incentivise more data collection than necessary. Furthermore, the principles of ‘accuracy’ and ‘storage limitation’ may be more difficult to fulfil with PETs, since – as in the case of federated learning – the process cannot usually be reversed and the raw input data is never stored.
Data subject rights
Beyond imposing obligations on data controllers, the GDPR also entitles individuals to a range of explicit rights with regard to data about them. These rights include the right to be informed, the right to access, and the right to erasure (Articles 12–23 GDPR). Many of these rights can currently not properly be exercised with PETs like federated learning, which is problematic. Again – as for complying with the GDPR’s principles while reducing negative outcomes like model bias, this is a balancing act on the part of the data controller. If it is not technically necessary, but the benefit provided to individuals is high, then it might be permissible to limit those rights, as long as sufficient safeguards are provided. This is, for example, the assessment of the Italian data protection authority (‘Garante’) with regard to the processing of personal data by ChatGPT, which currently does not enable a right to erasure. A similar argument will likely apply in the case of relevant PETs. Data Protection Impact Assessments Despite claims to the contrary, the GDPR does not generally embody a risk-based approach. Data controllers must seek to fulfil all obligations of the law, as they are stated in the legal text, and cannot just pick and choose. One of the few elements of the text that does require a good-faith assessment of risk is Data Protection Impact Assessments under Article 35 GDPR: ‘Where a type of processing in particular using new technologies and taking into account the nature, scope, context, and purposes of the processing, is likely to result in a high risk to … persons, the controller shall, prior to the processing, carry out an assessment of the impact of the envisaged processing operations…. A data protection impact assessment … shall in particular be required in the case of: … (b) processing on a large scale of special categories of data…’ (emphasis added) What is interesting from this Article is that the use of new technologies is explicitly singled out as a potentially high-risk activity, as is the large-scale processing of sensitive data (which includes health data). Since many applications of PETs use new technologies and aim to open up access to sensitive personal data (like health data), a thorough prior assessment of the risks in processing activities is likely necessary, alongside the implementation of adequate mitigation measures. If a high risk (such as incompatibility of processing with the GDPR’s principles, as discussed in the previous section) cannot be mitigated, prior consultation with the relevant data protection authority is likely necessary.
Conclusions
If PETs process personal data, then the GDPR applies; this includes applying ‘anonymisation’ techniques to personal data. The topic of joint controllership remains subject to substantial debate in the context of GDPR and will have important implications for legal compliance in PETs, including the obligations on third-party providers and facilitators of PETs. As it stands, PETs – like federated learning – provide little relief from GDPR obligations; indeed, they may even increase the burden on data controllers to ensure compliance with GDPR, including with its principles (e.g. lawfulness, fairness and transparency; accuracy; storage limitation; accountability) and data subject rights (e.g. the rights of access and to erasure). As technologies and attacks on them develop over time, organisations must keep up and continuously monitor their analysis of risk and legal compliance. If engaging in high-risk data processing (such as the use of new technologies or large-scale processing of sensitive personal data), a Data Protection Impact Assessment might need to be conducted and kept updated.