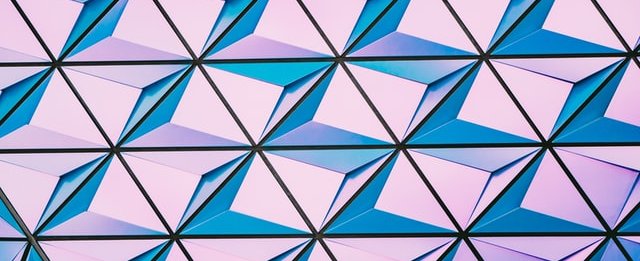
Researcher Konrad Kollnig spells out what federated analytics is, how it relates to federated learning and how the approach was used in Covid-19 contact tracing. This post builds on our research at the Open Data Institute (ODI) on how federated learning can be deployed to support responsible data stewardship. We want to understand the scope of such technologies to facilitate safe access to sensitive data. Ultimately this research will help developers, researchers, and organisations to follow best practice guidelines for data sharing and management.
When the Covid-19 pandemic first broke out, many had high hopes about contact-tracing apps to help prevent the spread of the virus. While the efficacy of these apps is still hotly debated and much beyond the scope of this article, these apps also present an interesting case study for the use of federated analytics (FA) at scale. FA is a new technique that promises to provide organisations with aggregate insights into the lives of individuals, while protecting the data of the individuals concerned. This article aims to provide more details on the FA technology in general, the interplay between FA and contact-tracking apps, and the lessons for decision-makers considering the use of FA in their projects.
Federated analytics
FA was first coined by Google in 2020. It emerged as a technique to complement Google’s use of Federated Learning (FL), a machine-learning technique whose potential the ODI is currently investigating in the context of public, educational and charitable aims. FA assumes that data is distributed across a set of network nodes, for example, individuals’ phones. Through a central server, data can be collected and aggregate statistics can be computed, both on the individual nodes and the central server. Ideally, the actual underlying data never gets shared with the central server.
Federated learning is a type of machine learning that does not send raw data to the machine-learning model, but instead brings the model to the data. The model is trained locally on each device, and the data never leaves its original location.The FA approach is particularly promising for applications like bias mitigation in machine learning, and for gaining completely new insights that could not be obtained otherwise. Crucially, FA is not a wholly new technique and is closely related to existing analytics techniques like distributed databases, which have been around for decades.
Privacy-preserving contact-tracing apps
Contact-tracing apps are probably well-known to most of us. They were available for both the Android and iOS mobile operating systems and allowed smartphones to track potential close contacts with confirmed Covid-19 infections. In England and Wales, contact-tracing was rolled out through the ‘NHS COVID-19’ app; other countries deployed similar apps. Most of these apps used the same technical protocol (Exposure Notification) which was collaboratively developed by Google and Apple. These companies themselves followed the blueprint by a team of academic researchers led by Carmela Troncoso from the Swiss Federal Institute of Technology Lausanne (EPFL) in Switzerland.
For tracking potential close contacts, the apps use a privacy-preserving approach. The emphasis on privacy was chosen, in part, to prevent the detailed tracking of individuals’ day-to-day lives by governments and other organisations – potentially even after the pandemic.
As part of this approach, a smartphone generates a new identifier every 15–20 minutes. This identifier is then shared through Bluetooth signals – over 15–20 minutes – with all nearby participating smartphones, which in turn keep track of what identifiers they saw and when. In addition to observed identifiers, participating smartphones also keep track of their own generated identifiers. Identifiers older than 14 days get discarded automatically.
If a participating individual now tests positive for Covid-19, this individual can then – voluntarily – upload that positive test result to a country-specific central server through their app. This report includes all the identifiers that this individual’s smartphone generated over the past 14 days. The central server, in turn, distributes these identifiers to all participating devices, which then check if they saw this identifier in the past 14 days and alert their user if they had – thereby suggesting a Covid-19 exposure.
To fed, or not to fed?
One can surely debate whether the case of contact-tracing apps is actually an FA use case. It clearly was never intended to be. And yet, it does bear many of the characterising features of FA: a central, coordinating node that derives insights from distributed nodes, that is, exposures to Covid-19. The actual data, that is, some permanent user identity, is never shared, but instead a short-lived identifier. Server-side aggregation is mostly limited to broadcasting exposure notifications. Yet, some countries, like France and Germany, also compute and share additional aggregate statistics about the number of received exposures and the number of participating devices, thereby giving some population-level insights into the spread of the virus. Due to the privacy-preserving approach, it is difficult for governments to generate more detailed insights.
Meanwhile, in other applications of FA, this technology might help build new surveillance architecture if privacy guarantees regarding the underlying data aren’t upheld. For example, it is probably fine to collect population level insights in the case that all affected individuals have given explicit, informed consent. Yet, the ethical case is less clear if data is collected without individuals having given such consent or when insights into small populations or single individuals are generated (especially if those subgroups are already marginalised or at a disadvantage).
There also remain concerns about what organisations will actually be tasked with making decisions over federations of data-generating nodes. There are the usual risks of advantages for incumbents and first movers. We already see this in applications like Google’s Private Compute Core on Android that enables Google to develop FA/FL applications on Android data but is not currently open to third-party developers. Those organisations that can already make far-ranging decisions about the technological infrastructure that we use might soon vastly increase their access to data, insights and power through approaches like FA and FL.
FA for public good?
For the short-term, FA remains a field of active research. Like in FL, many questions remain about the risks and benefits of the approach, including the actual privacy guarantees of FA and the potential competitive advantages of first movers and incumbents. Beyond the examples publicly documented by Google, there currently exist few examples of large-scale real-world deployment of FA. This is why this article focused on a slightly artificial case study that was never meant to be an FA application.
Get in touch
Because of the relative lack of previous work we would welcome any input and thoughts on the use of FA for public, charitable and educational aims. Please get in touch here.