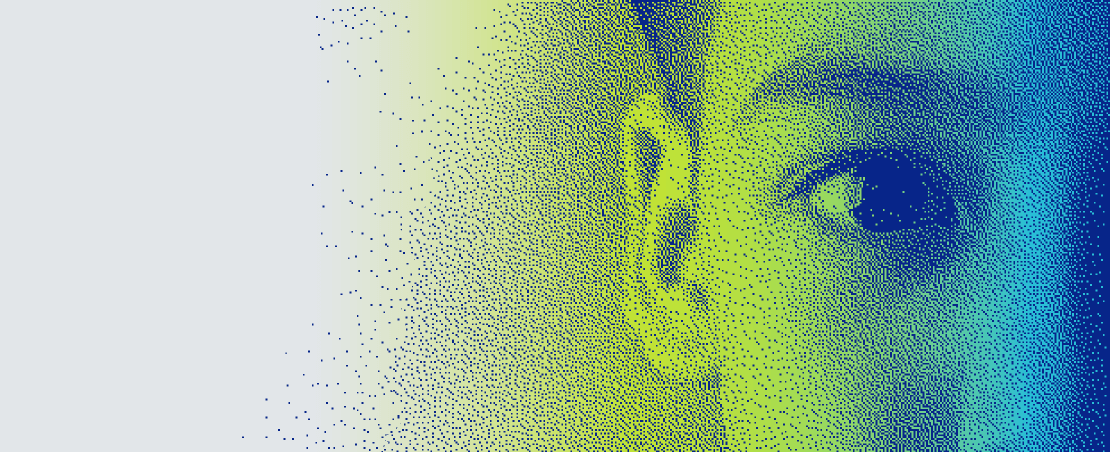
Artificial Intelligence (AI) may not be a new concept in the technology world, but the public release of ChatGPT a year ago marked a step change. The release gave anyone with access to the internet the ability to "talk" to an AI programme like ChatGPT, Claude, or Midjourney using text prompts rather than specialist language. It sparked an unprecedented wave of research, development, and policy-making that advanced our understanding of the technology and how it could be used ethically and equitably. It also sparked a lot of fear, uncertainty and doubt, including concerns about data privacy, the use of copyrighted content, and authenticity.
Beyond the hype, recent progress in foundational models (FMs) and their accelerated adoption by businesses and government bodies can bring significant opportunities for efficiencies, economic growth and innovation, but there are also significant risks of misinformation, job losses, discrimination, and social inequalities. Balancing these features requires an ecosystem view of AI, which acknowledges the role of data, computing, governance and regulation to move the field in the right direction over the next few years.
What is data-centric AI and why is it important?
Without data, there would be no AI – that applies to any form of AI, from deep learning, reasoning and planning to knowledge graphs. We need to look closer at the links between data and algorithms, drawing on approaches from multiple disciplines and engaging those directly affected by AI, as well as civic society. The latest wave of large language models (LLMs) and other FMs has disrupted how we think about many components of our data infrastructure: from the value of data we publish openly and the rights we hold on data, both individually and collectively, to the quality and governance of critical datasets. We are using the term ‘data-centric AI’ to advance our thinking in this space – the term was introduced a few years ago in the AI community to advocate for more attention to the data that AI engineers feed into their models. Expanding on the term, we use it to refer to the entire socio-technical data infrastructure of AI – this includes data assets, tools, standards, practices, and communities.
To deliver on AI safety and follow through on the commitments from the Bletchley Declaration, and other recent announcements and global regulations, we need to consider the data infrastructure of existing and future applications of AI. This goes beyond ongoing efforts to create benchmark datasets that, although useful for evaluating and comparing models, do not represent the vast scenarios in which AI is envisioned to be applied. As generative AI gains traction, there is a risk that, given the costs associated with good data practices, models will be trained and tested on synthetic or lower-quality data, leading – in time – to a degradation in performance and increasing the likelihood of harm. AI data infrastructure and better data practices should be adopted and mandated across industry, informed by the latest advances in data science and engineering, and supported by dedicated data institutions.
What our data-centric AI programme aims to achieve
Building on more than a decade of work creating open, trustworthy data ecosystems, the ODI has helped shift the AI narrative away from an exclusive focus on model development and use towards a wider understanding of the resources – and stakeholders – needed to enable sustainable and responsible technological development. The ODI acts as a key institution researching, connecting, and amplifying diverse ideas and approaches, developing and enabling best practices for data stewardship, and convening a wide range of stakeholders in the ecosystem, including startups, entrepreneurs, researchers, policy-makers and civic society, to help develop an AI data ecosystem grounded in responsible data practices.
Realising the potential of AI to benefit everyone and meet the commitments of the Bletchley Declaration, will require several essential steps in data-centric AI:
Make data AI-ready
- We need to enable and support the creation of high-quality AI datasets. Many AI datasets are small, synthetic, or not representative of a particular country, company or context. The result is benchmark saturation - models perform well on the data that is available, but worse when applied to solve real problems.
- Existing copyright, data protection, and worker rights must be respected when creating new AI datasets. We need more research to identify gaps in how these rights are currently protected or not in the datasets used by AI systems.
- Key AI datasets must be responsibly stewarded and governed. Some datasets are critical for specific sectors and need wise stewardship mechanisms to ensure they are used equitably and maintained to a high standard.
- Public-good datasets should be continuously supported as they boost innovation in many areas, including AI. A lot of progress in AI has been on the back of open datasets, but there is a danger people would stop contributing and investing in open data and most new data fed into AI models will be synthetic or of lower quality.
- Best practices in AI data assurance must be established and standardised. While some toolkits are emerging, there is limited guidance or regulation to assure datasets used in public services.
Make AI data accessible and usable
- We need to work with data holders to study critical datasets. Most datasets are poorly documented, which means that users find it difficult to understand their intended purpose, known use cases, and limitations.
- Fair and equitable data access must be mandated to develop AI use cases with big societal implications e.g. misinformation, climate, and infectious diseases.
- Data standards should be developed to reduce the cost of data operations and allow researchers and smaller businesses to build better AI data infrastructure.
- Safe access to datasets for startups and SMEs must be enabled, to boost responsible experimentation and innovation. This is one of the main roadblocks alongside access to computing and AI talent.
- The potential for new AI capabilities in making data more accessible, usable, and useful for everyone should be explored. There are opportunities for AI to automate or streamline processes that currently restrict or delay data sharing and use.
Make AI systems use data responsibly
- Explore mechanisms that improve understanding of data in the AI lifecycle. This includes exploring whether AI tech holders and downstream applications should be required to share information about data provenance and lineage to foster good data practices in the ecosystem and more thorough analysis of impacts.
- Invest in research and innovation to develop more protective and efficient AI models. This can aid the development of models that are less reliant on huge datasets, as well as models that rely less on massive amounts of data and do not trade off privacy and data protection for performance.
- Design, assess and promote more meaningful data licences that support publishers and users to implement good data practices in AI.
- Invest in creating more practical toolkits to inform new regulations and reduce compliance costs. Toolkits, case studies, and peer learning have a role to play in operationalising existing and emerging regulatory frameworks.
- Strengthen responsible AI practices through research, training and data literacy. AI engineers should be trained in responsible AI practices, and non-technical workers should have access to tools and training that help them understand the links between data and responsible AI.
The ODI is committed to advancing research and practice to implement these steps. This includes:
- frameworks to define and assess the value of AI data and AI business models;
- landscape reviews of emerging data-centric AI technologies such as federated learning and ML data assurance;
- innovation programmes supporting startups and artists in using data and AI responsibly to tackle grand challenges such as misinformation; ecology and international cooperation.
- learning courses on data ethics and AI and machine learning.
Building on this work, in the next few months, we will:
- map out the journey of data in AI model design, training, validation, testing and use to highlight common challenges in responsible data practices for AI
- study existing and emerging data stewardship and governance practices in the most popular AI datasets
- understand and describe the role of data-related challenges in AI incidents reports
- design computational approaches to assess the impact of open data sources on AI models' performance
- propose participatory approaches to data prompting as a means to help diverse audiences use generative AI tools to find and make sense of data
- establish policy priorities for a future AI bill in the UK, and other data legislation, building on the findings of the programme
There is much to do, and as technology and regulation move at lightning speed, we must prioritise this field of enquiry – and work quickly to transform ideas into action. We are excited to collaborate with Microsoft, the Industry Data for Society Partnership, King's College London, the University of Oxford, and many others to make progress and make a difference.
We are keen to hear from funders, partners and other organisations who are interested in helping to develop our programme of work. If you would like to contribute, talk about funding our work or challenge our thinking we welcome hearing from you.