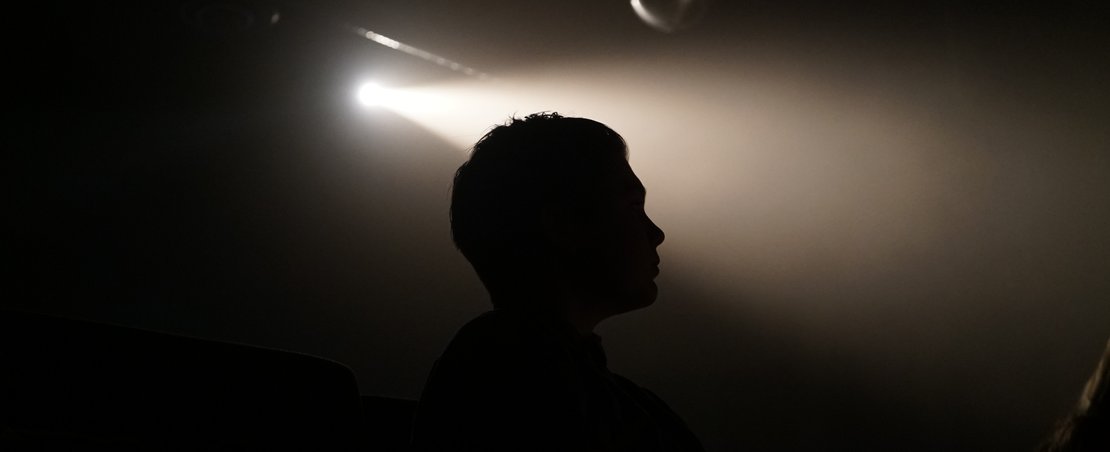
This blogpost is written by ODI Fellow Georgia Meyer You can get in touch with Georgia by emailing [email protected]
The UK government’s recently published Data Protection and Digital Information Bill (DPDI), the fate of which is now uncertain given recent turmoil, re-clarifies the already present General Data Protection Regulation (GDPR) position that anonymised personal data is not subject to data protection legislation. With increasing investment in Privacy Enhancing Technologies (PETs), it is important to consider what the implications are of a data economy increasingly fuelled by data processing that will arguably be divorced from oversight in many settings. If ‘privacy’ can, for argument’s sake, be ‘guaranteed’, what else is at stake?
This is the subject of my research as a new Research Fellow at the Open Data Institute (ODI) and as an MPhil/PhD student (Information Systems) at the London School of Economics and Political Science (LSE), supervised by Dr Edgar Whitley. In this blogpost, I set out some of the conceptual issues that may emerge from increased use of anonymised personal information by virtue of the increased deployment of PETs. The first part reviews the legal status of anonymised personal data in the DPDI (and GDPR). The second part addresses PETs in practice and their complex and contextual techno-organisational arrangements. The piece closes by teasing out some of the conceptual issues that may arise, like forfeited agency, from an increasing reliance on PETs and highlights efforts to re-stitch people back closer to processes that determine the nature of the data collected about them and the purposes to which it will be put.
If you are keen to discuss this further, please email me at [email protected].
The legal status of anonymised personal data
The DPDI, which had its first reading in the House of Commons on 18 July 2022, reasserted that anonymised data is not subject to controller and processor obligations (set out in Chapters 12-20 of the DPDI and all data). Under the GDPR, anonymised personal data is not stitched to identifiable data subjects and therefore their data subject rights (as set out in ICO guidance) are forfeited. This is because the information, once anonymised, does not relate to an ‘identifiable living individual’. In light of this, it is reasonable to expect that there won’t be significant changes to this definition despite the new secretary at the Department for Digital, Culture, Media and Sport (DCMS) signalling that the government plans to revise parts of the DPDI before it is reintroduced to the Commons. For a full list of definitions in the Bill, see Table 1.
The Bill sets out that, in order for information to be considered ‘personal data’, it must relate to an ‘identified or identifiable living individual’ (Clause 101). It goes on to outline that identification can happen directly or indirectly. In both cases the text stipulates that identification might occur by ‘reasonable means’ at the time of processing – taking account of the technology available at the time. Legal expert Chris Pounder has commented: ‘In other words, non-personal data and anonymous data are both free of any data protection concern (i.e. no obligations arise from the UK GDPR). The Bill’s objective is to widen the scope of these two categories by narrowing the scope of those data that are classified as “personal data”.’
Assuming that one or more PETs do work in practice and anonymise data derived from individuals, then the Bill stipulates that: ‘The legislation does not apply to non-personal or anonymous data.’ (Clause 101). There may be other governance mechanisms at work in a given context to ensure that data is used responsibly, that re-identification does not occur, and that the data is being used in the ways that were outlined when it was collected. However, this still points to a number of issues about what it might mean to have a data economy fuelled by anonymised information.
PETs in practice
PETs are a range of statistical, hardware and cryptographic techniques that are being designed to enable data processing that does not disclose information relating to identifiable living individuals. However they are by no means foolproof (re-identification has been shown to be possible in various cases) and there is a risk that their adoption could still result in exposure of the information of identifiable individuals. In spite of these concerns, PETs have long been seen as a potential means by which, and are indeed precipitating, greater data sharing in a ‘privacy' preserving manner. As such they are seen by many as a key part of ushering in the benefits of a data rich economy.
In a given context, it may well be that a number of PETs are employed during various stages of data collection, managing, sharing and analysis. For an overview of some of the most commonly used PETs see Table 2, and for a summary of the state of PETs see The Royal Society’s 2019 report ‘Protecting Privacy in Practice’ (an update is due for publishing in December 2022). Increased resources are being devoted to accelerate the deployment of PETs to remedy some of the risks associated with the use of personal data. Long heralded as a route to protecting privacy in healthcare and smart city settings, PETs are increasingly being seen as a potential route to inscribe ‘privacy by design’ into digital collection, processing and application architectures. A recently launched US-UK PETs challenge speaks to recent increases in attention their potential is receiving.
It is important to acknowledge that there are of course contexts within which the deployment of PETs can support important research and development breakthroughs (though of course defining importance is a contestable endeavour in and of itself). Professor Alison Noble is spearheading research with The Royal Society and The Alan Turing Institute to carefully steward this emergent terrain. Indeed, there will be situations where PETs are used in conjunction with additional elements of sound data governance, for example a data cooperative that stewards purposes, alongside a technique like homomorphic encryption which can resolve concerns over identification. The Royal Society 2019 report notes: ‘there is no technology that replaces the need for good governance and proper business practice relating to the use of data.’
At the same time it is also important to note developments in large-scale digital experimentation techniques that utilise enormous amounts of digital behavioural and psychographic data about people with an express aim to extrapolate ‘population level insights’. For example, see a recent conference at MIT (NB the DPDI Bill also stipulates that processing personal data for statistical purposes whereby the data generated is aggregate data is not subject to data protections). In such settings, by virtue of the fact that anonymisation permits insight generation whilst simultaneously protecting privacy (and hence circumnavigates data protection), it is prudent to begin to consider what else is at stake.
Forfeited agency
Considering this question goes to the very heart of epistemic issues about how knowledge gets produced, by whom and in service of whom/what. Moreover, what the reality-making properties that all ‘knowledge’, in turn, comes to have. One lens through which to consider the material impacts of technologies is that of performativity. There is an interesting and growing body of work looking to account for the impact of predictions on outcomes in models (see Mendler-Dünner et al 2022 and Perdomo et al 2021) calling into question the nature of the causal relations between variables and their purported relative impacts on outcomes. The relationships between models as companions to and / or creators of realities is a subject also explored extensively in Dr Erica Thompson’s forthcoming book 'Escape From Model Land' (out in December 2022).
Performativity is a useful lens through which to consider the potential negative consequences of anonymised personal information. It enables us to begin to think through how people come to be divorced from input into the reality-making properties which their data comes to have. The sensemaking frameworks which give data meaning are constructed. These constructed meanings, or even more fundamentally the choice of variables to classify and measure in the first place, in turn come to shape how we interpret reality. Recent work from the ODI’s Experimentalism and the Fourth Industrial Revolution project highlighted this in the ‘Asimov and data mirrors’ piece looking at post-Brexit data policy. Gavin Freeguard’s provocation in this chapter of the project asks precisely this: “How do the methods and metrics we use for data policy impact assessment shape data policy outcomes?”
Considering what types of decision-making are lost when anonymised personal information circumnavigates legal protections raises questions about the robustness of the privacy paradigm to take account of the relational nature of data. Investigating the relational nature of data is the ambition of Dr Jeni Tennison’s, former CEO at the ODI, new organisation Connected by data, whose mission is to: ‘put community at the centre of data narratives, practices and policies by advocating for collective and open data governance’.
There is a slight shift in emphasis from language around data rights – typically used to frame sound data governance, to embed community within narratives and practices. When data campaigning that advocates for people to have more control over the purposes to which ‘their’ data will be put is framed around data as owned and with rights, it can miss the relational aspect of data. Much of the language of data rights is enshrined in data protection legislation in obligations like purpose limitation, data minimisation, the right to be forgotten etc. Though a conversation about the extent to which an economic and legal lexicon around stable, classifiable, ownable and valuable ‘personal data’ is compatible with a relational concept of data, and emerging ideas about relational ethics, remains.
Connected by data’s mission to put communities at the centre of data practices, and foreground relational impacts from data use, is also reflected in the enormous range of work around more localised community-centred data arrangements that place emphasis on collective agency in determining collection and purposes (which comprises initiatives around personal and non-personal data). See the work of Aapti Institute’s Data Economy Lab (especially the Data Stewardship Playbook and Data Stewardship Navigator), Mozilla Foundation’s Database of Initiatives: Alternative Data Governance in Practice, the ODI’s’s Data Institutions Register, and Pollicy’s work on data governance and rights.
What ties these examples together is the focus on narrowing the space between the people from whom data is collected and the decision-making processes about the purposes to which that data will be put, often articulated in terms of dimensions of value beyond simply market measures. So considering what the risks of a data economy fueled by anonymised data are could start with recognising that this scenario sets up an opposite force: one that increasingly separates individual and collective decision-making processes from how data is used in practice.
Growing the conversation
It strikes me that some of the consequences of this emergent terrain will start to pull at the saliency of various frameworks for ‘protecting’ data, like conceptual robustness of privacy for example. Moreover, that focused attention will need to be devoted to thinking through how various PETs unfold in practice, with some of the above in mind.
I’m pursuing both of these themes in my research and would be delighted to hear from anyone else working in this technical field and PhD or postdoc researchers. It would be great to share ideas and find ways to collaborate on where and how conceptual issues meet technical and legal challenges.
Get in touch by emailing [email protected]
Appendix
Table 1 – Definitions in the Data Protection and Digital Information Bill (DPDI)
[table id=83 /]
Table 2 – Most commonly used Privacy Enhancing Technologies (PETs)
[table id=84 /]